Embedded AI And Machine Learning Adding New Advancements In Tech Space
15 Jan, 20213 MinsThroughout the most recent years, as sensor and MCU costs dove and shipped volumes have gone...
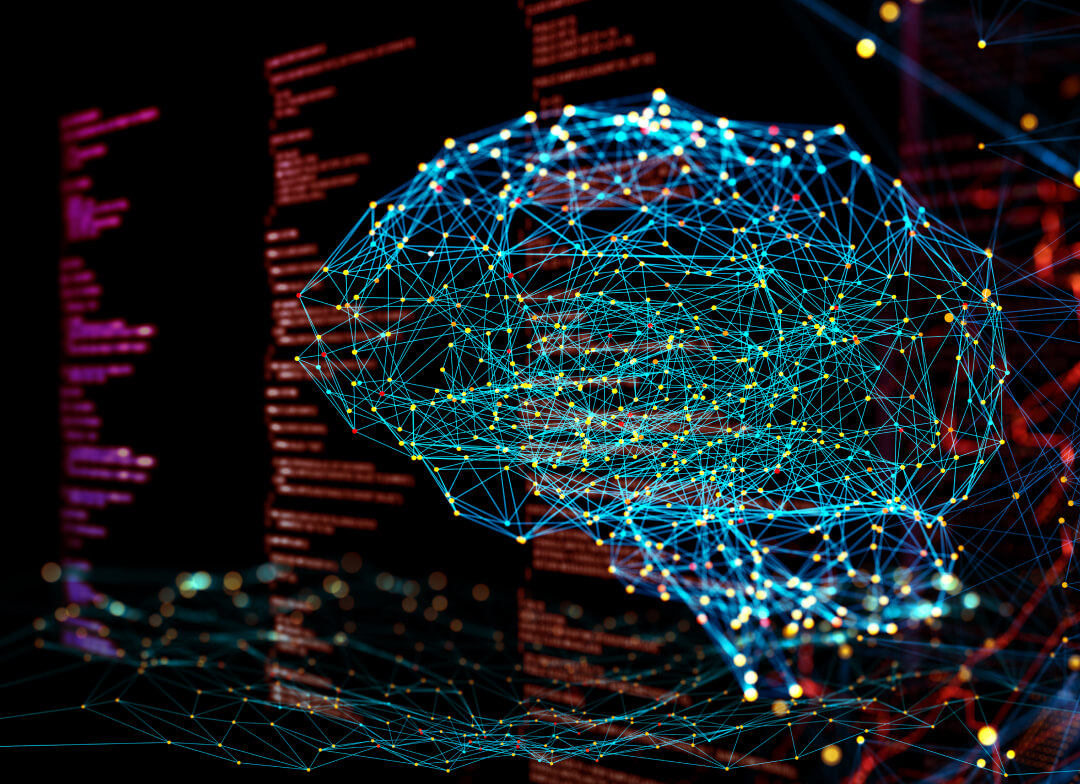
Throughout the most recent years, as sensor and MCU costs dove and shipped volumes have gone through the roof, an ever-increasing number of organizations have attempted to exploit by adding sensor-driven embedded AI to their products.
Automotive is driving the trend– the average non-autonomous vehicle presently has 100 sensors, sending information to 30-50 microcontrollers that run about 1m lines of code and create 1TB of data per vehicle every day. Extravagance vehicles may have twice the same number of, and autonomous vehicles increase the sensor check significantly more drastically.
Yet, it’s not simply an automotive trend. Industrial equipment is turning out to be progressively “brilliant” as creators of rotating, reciprocating and other types of equipment rush to add usefulness for condition monitoring and predictive support, and a huge number of new consumer products from toothbrushes, to vacuum cleaners, to fitness monitors add instrumentation and “smarts”.
An ever-increasing number of smart devices are being introduced each month. We are now at a point where artificial intelligence and machine learning in its exceptionally essential structure has discovered its way into the core of embedded devices. For example, smart home lighting systems that automatically turn on and off depend on whether anybody is available in the room. By all accounts, the system doesn’t look excessively stylish. Yet, when you consider everything, you understand that the system is really settling on choices all alone. In view of the contribution from the sensor, the microcontroller/SOC concludes if to turn on the light or not.
To do all of this simultaneously, defeating variety to achieve troublesome detections in real-time, at the edge, inside the vital limitations isn’t at all simple. In any case, with current tools, integrating new options for machine learning for signals (like Reality AI) it is getting simpler.
They can regularly achieve detections that escape traditional engineering models. They do this by making significantly more productive and compelling utilization of data to conquer variation. Where traditional engineering approaches will ordinarily be founded on a physical model, utilizing data to appraise parameters, machine learning approaches can adapt autonomously of those models. They figure out how to recognize signatures straightforwardly from the raw information and utilize the mechanics of machine learning (mathematics) to isolate targets from non-targets without depending on physical science.
There are a lot of different regions where the convergence of machine learning and embedded systems will prompt great opportunities. Healthcare, for example, is now receiving the rewards of putting resources into AI technology. The Internet of Things or IoT will likewise profit enormously from the introduction of artificial intelligence. We will have smart automation solutions that will prompt energy savings, cost proficiency as well as the end of human blunder.
Forecasting is at the center of so many ML/AI conversations as organizations hope to use neural networks and deep learning to conjecture time series data. The worth is the capacity to ingest information and quickly acknowledge insight into how it changes the long-term outlook. Further, a large part of the circumstance relies upon the global supply chain, which makes improvements significantly harder to precisely project.
Probably the most unsafe positions in production lines are as of now being dealt by machines. Because of the advancement in embedded electronics and industrial automation, we have ground-breaking microcontrollers running the whole mechanical production systems in assembling plants. However, the majority of these machines are not exactly completely automatic and still require a type of human intercession. In any case, the time will come when the introduction of machine learning will help engineers concoct truly intelligent machines that can work with zero human mediation.
Source: Analytics Insight